In order to commence this discussion, we need to first cover the basics of Appen's business model (the core of what they do) so that the challenges can be understood.
I'd encourage people to get a basic grounding in machine learning - there are many visual examples that will explain this in layman's terms. Search on YouTube or one of the learning platforms.
Appen's customers produce these learning algorithms. An example might be, a person (learning algorithm) learns by reading books in a local library. The only thing that they know about is from those books. The quality of those books determines how much knowledge is learnt. It's not a matter of just providing more books. Look at the internet - people have access to more information than every before and we've arguably become less intelligent. The quality of books is much more important than the volume of books.
Appen's customers (who produce the learning algorithms) typically know a good selection of books to teach a particular subject. Think of these subjects as applications (speech, language, vision, search, etc.). Each of these subjects are incredibly different. The fundamentals (of taking a learning algorithm, applying training data and tuning to get a result) haven't changed. However the domain specific knowledge of these applications is very important, in order to know what topics to cover in the books. I have experience in some of these, however I'm completely blind when it comes to other application areas.
So how do these books get created? In the early days, it was the expert who wrote the algorithms who would handcraft these books. They know the concepts that they need covered and could explain in great detail. This made training data very expensive to produce. Cheaper labour methods were introduced, such as interns or junior staff, who had some knowledge of the algorithms and could still maintain sufficient level of quality. Crowdsourcing techniques started to be applied in order to get much larger volumes of data. This basically involves breaking down the the tasks into tiny sub-tasks that can be performed with a very low level of knowledge. In order to maintain a workforce, you need the tasks to be so easy that they aren't viewed with much value. In order to maintain quality, it's usually better to maintain the same workforce. However, by doing so you start to introduce bias.
It's impossible to have a learning algorithm that doesn't have a certain level of bias. Bias exists in everything that we do. That's why I mentioned previously that domain knowledge is incredibly important. I know in depth about 2 particular domains that are related. This knowledge allows the customers to outline the type of training data that they need. Some customers won't have this level of understanding. They may have people capable of implementing the learning algorithms but not actually understanding the finer details of how they were designed and what they need to operate effectively.
There is another type of bias that you will read about and that's to do with the influence that the customer's product has on an outcome in society. For example, if an HR department was using machine learning to determine what candidates to hire, it may be emphasise stereotypes that exist in the training data that are not socially acceptable today. e.g. an engineering role may favour men, nursing may favour women, etc. I've touched on this previously, but as technology causes disruption into new areas typically the laws and regulation take a while to follow through. We are starting to see some of that at the moment.
You can think of the creation of a human-annotated dataset as a production line. There are people doing very simple small tasks. Some sections of the production line use robots, due to the increased efficiency, higher level of accuracy, etc. These robots are essentially another use of learning algorithms. A large component of the work is project management and quality control. Some of these functions can be automated however the general concept of maintaining a human workforce remains the same.
In relevant to the relevant areas that you highlighted in Appen;
1) Relevance vs domain specific.
These are the domain specific applications (e.g. speech, language, vision, search, etc.). The customers that they have determine the volume of work in these areas. Self-driving vehicles obviously caused a large increase in vision. In order to create quality training data, Appen needs expertise in these areas. If a new area emerges, they need to adapt.
2) - 5) Government, China, Enterprise, Global
These are just market segments. The only one that I see as a particular challenge is China. China has made incredible advances in AI. Their style of government allows them to motivate people differently to return to China and dedicate their skills and experience. China understands the importance of having a long term strategy in AI. If you want to be an expert in something, do you think it's better to learn everything about it or just focus on a particular section? China has access to very cheap labour. Once they understand the work that is required, I'm sure they will figure out a cheaper way of annotating data.
6) Human-centric data annotation vs automated methods
From my perspective, we will always require a human-in-the-loop in order to maintain quality. To clarify, Appen's customers will already have mass amounts of their own data that they've used and introduced all kinds of bias. There is a threat that automated methods will decrease the value of human-centric data annotation. However, this is also an opportunity for Appen to enhance their knowledge in these areas and use more automation in their production lines. To give a relevant example, Tesla were a large customer of Appen. They need mass amounts of training data annotated, so they focused on working out how they could automate this. It's not a matter of A) vs B). It's a natural progression that this industry continuously faces. Both customers and vendors need to keep up-to-date.
7) Ethical AI or unbiased solutions
This will just be another product area for Appen. Once regulation comes in place, this mandates a timeframe for when their customers need to react. If they are large, Enterprise/Government, etc. they are typically incredibly slow to react so this results in more work for companies such as Appen.
8) Regulatory landscape
Are you referring to regulation of their workforce? Some people view crowdsourced learning as a form of slavery. Rider sharing & Food Delivery platforms often make this look glamorous but it's focused on exploiting cheap labour.
USA & EU are likely to have regulations around this type of work in the future.
However, countries such as China will be happy to take whatever production lines are too slow and expensive due to our regulations.
This is still incredibly high level, but I hope you understand why given that the base level of understanding is still quite low.
- Forums
- ASX - By Stock
- APX
- brave souls rewarded today
APX
appen limited
Add to My Watchlist
4.35%
!
$1.20
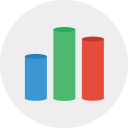
brave souls rewarded today, page-72
Featured News
Add to My Watchlist
What is My Watchlist?
A personalised tool to help users track selected stocks. Delivering real-time notifications on price updates, announcements, and performance stats on each to help make informed investment decisions.
|
|||||
Last
$1.20 |
Change
0.050(4.35%) |
Mkt cap ! $317.8M |
Open | High | Low | Value | Volume |
$1.13 | $1.21 | $1.13 | $10.06M | 8.559M |
Buyers (Bids)
No. | Vol. | Price($) |
---|---|---|
1 | 6994 | $1.20 |
Sellers (Offers)
Price($) | Vol. | No. |
---|---|---|
$1.21 | 123190 | 6 |
View Market Depth
No. | Vol. | Price($) |
---|---|---|
1 | 6994 | 1.200 |
4 | 101703 | 1.195 |
4 | 8000 | 1.190 |
3 | 26300 | 1.185 |
9 | 27127 | 1.180 |
Price($) | Vol. | No. |
---|---|---|
1.210 | 123190 | 6 |
1.215 | 103855 | 4 |
1.220 | 55488 | 8 |
1.225 | 1817 | 1 |
1.230 | 11469 | 3 |
Last trade - 16.10pm 16/07/2025 (20 minute delay) ? |
Featured News
APX (ASX) Chart |
The Watchlist
WCE
WEST COAST SILVER LIMITED
Bruce Garlick, Executive Chairman
Bruce Garlick
Executive Chairman
Previous Video
Next Video
SPONSORED BY The Market Online