There can be no excuse for folks to educate themselves 8tey, u hand it on a plate.
- Forums
- ASX - By Stock
- 4DS
- 4DS - Anything but Charting
4DS
4ds memory limited
Add to My Watchlist
3.70%
!
2.8¢
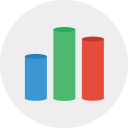
4DS - Anything but Charting, page-24557
Featured News
Add to My Watchlist
What is My Watchlist?
A personalised tool to help users track selected stocks. Delivering real-time notifications on price updates, announcements, and performance stats on each to help make informed investment decisions.
|
|||||
Last
2.8¢ |
Change
0.001(3.70%) |
Mkt cap ! $57.70M |
Open | High | Low | Value | Volume |
2.7¢ | 2.8¢ | 2.7¢ | $63.2K | 2.287M |
Buyers (Bids)
No. | Vol. | Price($) |
---|---|---|
9 | 591158 | 2.7¢ |
Sellers (Offers)
Price($) | Vol. | No. |
---|---|---|
2.8¢ | 977369 | 4 |
View Market Depth
No. | Vol. | Price($) |
---|---|---|
9 | 591158 | 0.027 |
25 | 5587769 | 0.026 |
29 | 3982485 | 0.025 |
17 | 2268964 | 0.024 |
9 | 2162824 | 0.023 |
Price($) | Vol. | No. |
---|---|---|
0.028 | 400000 | 2 |
0.029 | 237772 | 4 |
0.030 | 1400000 | 5 |
0.031 | 1810000 | 2 |
0.032 | 1849900 | 8 |
Last trade - 16.10pm 16/07/2025 (20 minute delay) ? |
Featured News
4DS (ASX) Chart |
The Watchlist
LU7
LITHIUM UNIVERSE LIMITED
Iggy Tan, Executive Chairman
Iggy Tan
Executive Chairman
Previous Video
Next Video
SPONSORED BY The Market Online