These researchers who are independent of Brainchip Inc appear to have a very different opinion to MF where Blackwall style computing and spiking neural network computing is concerned. Indeed they see a future where SNNs that have mastered Sequential learning will replace the GPU for deployed GPT4.[Submitted on 14 Dec 2023]Learning Long Sequences in Spiking Neural Networks
Matei Ioan Stan (The University of Manchester), Oliver Rhodes (The University of Manchester)"This paves the way for deploying SSM-based SNNs to neuromorphic hardware, which could drastically reduce the energy requirements of sequential models. Taking into consideration the efficient scaling of computations with respect to sequence length, SSM-based SNNs could have the potential to replace current solutions such as GPU-deployed GPT4 [OpenAI, 2023]"
As some here clearly missed it this is what Brainchip unleashed last year when announcing AKIDA 2.0:“Introducing TENNs
In March this year, we announced a new neural network architecture we call Temporal Event-based Neural Networks (TENNs). These are lightweight neural networks that excel at processing temporal data much more efficiently than traditional Recurrent Neural Networks (RNN) like Long Short-Term Memory (LSRM), or Gated Recurrent Units (GRU). These models often require substantially fewer parameters and orders of magnitude fewer operations to achieve equivalent or better accuracy compared to traditional models. The second generation Akida neural processor platform has also added extremely efficient 3-dimensional (3D) convolution functions that can therefore perform compute-heavy tasks on devices with limited memory and battery resources.
This feat is accomplished by combining the convolution in the 2D spatial domain with that of the 1D time domain, resulting in fewer parameters and fewer multiply-accumulate (MACs) operations per inference than previous networks. This reduced need for computation leads to a substantial reduction in the energy or power draw, making TENNs ideal for ultra-low-power Edge devices.
Specialized Processing
We have published our research about the advantages of TENNs when they are applied to spatiotemporal data like video from traditional frame-based cameras and events from dynamic vision sensors. TENNs can enable higher quality video object detection in tens of milliwatts.
TENNs are equally suitable for processing purely temporal data, like raw audio from microphones and vital signs from health monitors. In these situations, TENNs can minimize the need for expensive DSP or filtering hardware thereby reducing silicon footprint, energy draw as well as Bill of Materials (BOM) cost. This clears the path for much more compact form factor devices such as more advanced hearables, wearables or even new, embeddable medical devices that can be sustained through energy-harvesting.
Since TENNs can operate on data from different sensors, they radically improve analytical decision-making and intelligence for multi-sensor environments in real-time.
The Akida platform still includes Edge learning capabilities for classifier networks. This complements TENNs to enable a new generation of smart, secure Edge devices that far exceeds what has been possible so far in power envelopes that are measured in milliwatts or microwatts.”
Take the time to listen again to the presentation by Dr. Anthony Lewis Chief Technical Officer at Brainchip in this years joint podcast with the CEO Sean Hehir and his comments regarding LLM:
My opinion only DYOR
Fact FinderSpiking neural networks (SNNs) take inspiration from the brain to enable energy-efficient computations. Since the advent of Transformers, SNNs have struggled to compete with artificial networks on modern sequential tasks, as they inherit limitations from recurrent neural networks (RNNs), with the added challenge of training with non-differentiable binary spiking activations. However, a recent renewed interest in efficient alternatives to Transformers has given rise to state-of-the-art recurrent architectures named state space models (SSMs). This work systematically investigates, for the first time, the intersection of state-of-the-art SSMs with SNNs for long-range sequence modelling. Results suggest that SSM-based SNNs can outperform the Transformer on all tasks of a well-established long-range sequence modelling benchmark. It is also shown that SSM-based SNNs can outperform current state-of-the-art SNNs with fewer parameters on sequential image classification. Finally, a novel feature mixing layer is introduced, improving SNN accuracy while challenging assumptions about the role of binary activations in SNNs. This work paves the way for deploying powerful SSM-based architectures, such as large language models, to neuromorphic hardware for energy-efficient long-range sequence modelling.
- Forums
- ASX - By Stock
- BRN
- Nvidia
BRN
brainchip holdings ltd
Add to My Watchlist
2.44%
!
20.0¢
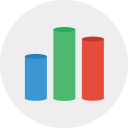
Nvidia, page-49
Featured News
Add to My Watchlist
What is My Watchlist?
A personalised tool to help users track selected stocks. Delivering real-time notifications on price updates, announcements, and performance stats on each to help make informed investment decisions.
|
|||||
Last
20.0¢ |
Change
-0.005(2.44%) |
Mkt cap ! $405.0M |
Open | High | Low | Value | Volume |
20.5¢ | 20.5¢ | 20.0¢ | $406.0K | 2.012M |
Buyers (Bids)
No. | Vol. | Price($) |
---|---|---|
23 | 559479 | 20.0¢ |
Sellers (Offers)
Price($) | Vol. | No. |
---|---|---|
20.5¢ | 2087426 | 37 |
View Market Depth
No. | Vol. | Price($) |
---|---|---|
12 | 262016 | 0.200 |
38 | 2475083 | 0.195 |
74 | 2169655 | 0.190 |
49 | 1905401 | 0.185 |
71 | 1783990 | 0.180 |
Price($) | Vol. | No. |
---|---|---|
0.205 | 1851568 | 35 |
0.210 | 1146130 | 25 |
0.215 | 666226 | 19 |
0.220 | 1927120 | 45 |
0.225 | 534126 | 9 |
Last trade - 16.14pm 11/07/2025 (20 minute delay) ? |
Featured News
BRN (ASX) Chart |
The Watchlist
VMM
VIRIDIS MINING AND MINERALS LIMITED
Rafael Moreno, CEO
Rafael Moreno
CEO
SPONSORED BY The Market Online