The following paper supports the unique importance of Brainchip’s patented One Shot Learning first revealed in AKD1000. I have extracted a few paragraphs to give an insight into what these researchers have been able to achieve using AKD1000 which will be essential if automotive makers are to successfully develop mass consumption autonomous automobiles where energy efficiency, customisation to each driver, accuracy, continuous improvement and latency are critical:
Few-Shot Transfer Learning for Individualized Braking Intent Detection on Neuromorphic HardwareNathan A. Lutes1, Venkata Sriram Siddhardh Nadendla2,∗, K. Krishnamurthy11 Department of Mechanical and Aerospace Engineering, Missouri University of Science and Technology, 400 W. 13th Street, Rolla, MO, USA, 654092 Department of Computer Science, Missouri University of Science and Technology, 500 W. 15th Street, Rolla, MO, USA, 65409.∗ Author to whom any correspondence should be addressed.Abstract.Objective:This work explores use of a few-shot transfer learning method to train and implement a convolutional spiking neural network (CSNN) on a BrainChip Akida AKD1000 neuromorphic system-on-chip for developing individual-level, insteadof traditionally used group-level, models using electroencephalographic data. The efficacy of the method is studied on an advanced driver assist system related task of predicting braking intention. Approach: Data are collected from participants operating an NVIDIA JetBot on a testbed simulating urban streets for three different scenarios. Participants receive a braking indicator in the form of: 1) an audio countdown in a nominal baseline, stress-free environment; 2) an audio countdown in an environment with added elements of physical fatigue and active cognitive distraction; 3) a visual cue given through stoplights in a stress-free environment. These datasets are then used to develop individual-level models from group-level models using a few-shot transfer learning method, which involves: 1) creating a group-level model by training a CNN on group-level data followed by quantization and recouping any performance loss using quantization-aware retraining; 2) converting the CNN to be compatible with Akida AKD1000 processor; and 3) training the final decision layer on individual-level datasets to create individual-customized models using an online Akida edge-learning algorithm. Main Results: Efficacy of the above methodology to develop individual specific braking intention predictive models by rapidly adapting the group-level model in as few as three training epochs while achieving at least 90% accuracy, true positive rate and true negative rate is presented. Further, results show an energy reduction of over 97% with only a 1.3× increase in latency when using the Akida AKD1000 processor for network inference compared to an Intel Xeon CPU. Similar results were obtained in a subsequent ablation study using a subset of five out of 19 channels. Significance: Especially relevant to real-time applications, this work presents an energy-efficient, few- shot transfer learning method that is implemented on a neuromorphic processor capable of training a CSNN as new data becomes available, operating conditions change, or to customize group-level models to yield personalized models unique to each individual…1.2. ContributionThe main contribution of this work is to present an energy efficient, few-shot transfer learning method of training a deep SNN using BrainChip Akida AKD1000 for development of individual-level cognitive models and demonstrate the efficacy of this method on an EEG-based ADAS application to detect anticipatory brain potentials at the individual level. Past EEG studies tend to focus on group-level models aimed at generalizing to a large population. However, this scale of model can be less than ideal because individuals exhibit different responses to the same task. Therefore, it is imperative to develop and use models customized to each individual, especially in real- time applications. The use of a neuromorphic processor is also novel in the context of ADAS literature…5. Conclusion…… The method presented demonstrates that individual-level models could be quickly created with a small amount of data, achieving greater than 90% scores across all three classification performance metrics in a few shots (three epochs) on average for both the ACS and FCAS. This demonstrated the efficacy of the method for different participants operating under non-ideal conditions and using realistic driving cues and further suggests that a reduced data acquisition scheme might be feasible in the field. Furthermore, the applicability to energy-constrained systems was demonstrated through comparison of the inference energy consumed with a very powerful CPU in which the Akida processor offered energy savings of 97% or greater. The Akida processor was also shown to be competitive in inference latency compared to the CPU.
My opinion only DYOR
Fact Finder
- Forums
- ASX - By Stock
- BRN
- 2024 BrainChip Discussion
BRN
brainchip holdings ltd
Add to My Watchlist
0.00%
!
22.5¢
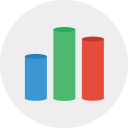
2024 BrainChip Discussion, page-8042
Featured News
Add to My Watchlist
What is My Watchlist?
A personalised tool to help users track selected stocks. Delivering real-time notifications on price updates, announcements, and performance stats on each to help make informed investment decisions.
|
|||||
Last
22.5¢ |
Change
0.000(0.00%) |
Mkt cap ! $455.7M |
Open | High | Low | Value | Volume |
22.5¢ | 23.0¢ | 22.0¢ | $1.378M | 6.134M |
Buyers (Bids)
No. | Vol. | Price($) |
---|---|---|
27 | 917929 | 22.0¢ |
Sellers (Offers)
Price($) | Vol. | No. |
---|---|---|
22.5¢ | 206324 | 4 |
View Market Depth
No. | Vol. | Price($) |
---|---|---|
27 | 917929 | 0.220 |
16 | 762651 | 0.215 |
28 | 1111176 | 0.210 |
21 | 825253 | 0.205 |
61 | 1899586 | 0.200 |
Price($) | Vol. | No. |
---|---|---|
0.225 | 206324 | 4 |
0.230 | 1326997 | 50 |
0.235 | 629461 | 18 |
0.240 | 539395 | 21 |
0.245 | 1146692 | 14 |
Last trade - 16.10pm 21/07/2025 (20 minute delay) ? |
Featured News
BRN (ASX) Chart |