Hi rayz
Well you have not lost your touch. Another golden nugget. I have highlighted the paragraph which points out just how far ahead Brainchip is in the race to true Artificial General Intelligence. Noting that DARPA has access to all the worlds secrets and everything that Intel, Nvidia, SpaceX etc; has to offer they make the point that no neural network has what AKIDA technology has and just think Versions 2 & 3 are about to be made real as well:
Artificial Intelligence Exploration (AIE) Opportunity
DARPA-PA-20-02-03 Time-Aware Machine Intelligence (TAMI)
I. Opportunity Description
The Defense Advanced Research Projects Agency (DARPA) is issuing an Artificial Intelligence
Exploration (AIE) Opportunity inviting submissions of innovative basic or applied research
concepts in the technical domain of time-aware neural network architectures that introduce a
meta-learning capability into data-driven machine learning to enable time-based machine
cognition and intelligence. This AIE Opportunity is issued under the Program Announcement for
AIE, DARPA-PA-20-02. All awards will be made in the form of an Other Transaction (OT) for
prototype project. The total award value for the combined Phase 1 base and Phase 2 option is
limited to $1,000,000. This total award value includes Government funding and performer cost
share, if required or if proposed.
To view the original DARPA Program Announcement for AIE, visit beta.SAM.gov (formerly
FedBizOps) under solicitation number DARPA-PA-20-02:
https://beta.sam.gov/opp/667875ba2f464ccfa38688ea1a718fe7/view?keywords=DARPA-PA-20-
02&sort=-relevance&index=opp&is_active=true&page=1
A. Introduction
The Time-Aware Machine Intelligence (TAMI) AIE Opportunity will develop new time-aware
neural network architectures that introduce a meta-learning capability into machine learning.
This meta-learning will enable a neural network to capture the time-dependencies of its encoded
knowledge.
As a neural network learns knowledge about the world and encodes it in its internal weights,
some learned weights may encode knowledge whose activation should be conditioned based on
time. Examples of such time dependencies are the weights mapped to the appearance features of
a person in a convolutional neural network (CNN) for object recognition or the weights mapped
to the dynamic features of a person’s gait in a recurrent neural network (RNN) for activity
recognition – both are only valid for a finite interval of time. Current neural networks do not
explicitly model the inherent time characteristics of their encoded knowledge. Consequently,
state-of-the-art (SOA) machine learning does not have the expressive capability to reason with
encoded knowledge using time. An inference network, for example, cannot discount activations
of weights for time-conditioned knowledge as features become less relevant over time. This lack
of time dimension in a network’s knowledge encoding limits the “shelf life” of the systems,
leading to outdated decisions and requiring frequent and costly retraining to optimize
performance.
TAMI’s vision is for an AI system to develop a detailed self-understanding of the time
dimensions of its learned knowledge and eventually be able to “think in and about time” when
exercising its learned task knowledge in task performance.
TAMI draws inspiration from ongoing research on time processing mechanisms in human brains.
A large number of computational models have been introduced in computational neuroscience to
explain time perception mechanisms in the brain. TAMI will go a step further from such research
to develop and prototype concrete computational models. TAMI will leverage the latest research
on meta-learning in neural networks. Recent neural network models with augmented memory
capacities are possible starting points for investigating the meta-learning of time dependencies.
DARPA-PA-20-02
Additionally, neural network based temporal knowledge graph modeling may provide
mechanisms to infer hidden temporal relations of entities.
B. Objective/Scope
TAMI seeks to develop a new class of neural network architectures that incorporate an explicit
time dimension as a fundamental building block for network knowledge representation. TAMI
will develop new time-modeling components into such networks and investigate learning
paradigms that can simultaneously learn task knowledge and be able to develop a self-reference
to the details of the time dependencies of its knowledge encoding as meta-knowledge.
As motivation for the TAMI vision, consider neural networks designed for inference. Such
neural networks derive abstract task knowledge from the analysis of a large number of data
samples. Each data sample exists only in a specific time. For example, features given by a
vehicle data sample are associated with that specific vehicle’s age (e.g., rust and dents) and,
therefore, are explicitly dependent on time. Neural networks incorporate such information as
static activation weights; however, using the example above, the activation of these weights
should ideally be conditioned on time.
Since neural network’s knowledge encoding is a composite from features of many data samples,
the time properties of the encoded knowledge in a neural network are complex functions of the
time properties of the data from which the knowledge was built. Simply encoding timestamps or
aggregating learning data according to time duration is insufficient, as machine learning cannot
know beforehand which aspects of its encoded knowledge remain time conditioned.
Furthermore, other time-related properties exist in a neural network’s knowledge encoding,
dependent on the type of task learned. A new learning mechanism is needed to enable a selfawareness of the complex time-conditioned property of neural networks’ knowledge encoding.
TAMI’s objective differs from other machine learning research on machine time perception and
temporal knowledge modeling where the focus is on modeling the time properties in the source
data and encoding them in the neural network model. TAMI focuses on modeling the time
property of its own learning. In other words, TAMI will develop a form of meta-learning into
neural networks.
My opinion only DYOR
- Forums
- ASX - By Stock
- 2020 BRN Discussion
BRN
brainchip holdings ltd
Add to My Watchlist
1.22%
!
20.8¢
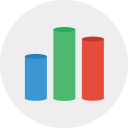
Hi rayzWell you have not lost your touch. Another golden nugget....
Featured News
Add to My Watchlist
What is My Watchlist?
A personalised tool to help users track selected stocks. Delivering real-time notifications on price updates, announcements, and performance stats on each to help make informed investment decisions.
|
|||||
Last
20.8¢ |
Change
0.003(1.22%) |
Mkt cap ! $415.2M |
Open | High | Low | Value | Volume |
20.5¢ | 21.0¢ | 20.3¢ | $756.3K | 3.688M |
Buyers (Bids)
No. | Vol. | Price($) |
---|---|---|
10 | 42874 | 20.5¢ |
Sellers (Offers)
Price($) | Vol. | No. |
---|---|---|
21.0¢ | 1732636 | 34 |
View Market Depth
No. | Vol. | Price($) |
---|---|---|
75 | 2490353 | 0.200 |
38 | 1382204 | 0.195 |
64 | 2059608 | 0.190 |
45 | 1583398 | 0.185 |
69 | 1261218 | 0.180 |
Price($) | Vol. | No. |
---|---|---|
0.205 | 172800 | 11 |
0.210 | 1671346 | 32 |
0.215 | 1511458 | 22 |
0.220 | 2087670 | 51 |
0.225 | 896366 | 15 |
Last trade - 13.08pm 04/07/2025 (20 minute delay) ? |
Featured News
BRN (ASX) Chart |
The Watchlist
NUZ
NEURIZON THERAPEUTICS LIMITED
Dr Michael Thurn, CEO & MD
Dr Michael Thurn
CEO & MD
SPONSORED BY The Market Online