At the same conference the following further two papers were presented which might be of interest:
This one because of the TCS paper by Dr. Arpan Pal:
6:50PM ECG-based Heartbeat Classification in Neuromorphic Hardware [#19235] Federico Corradi, Pande Sandeep, Jan Stuijt, Ning Qiao, Siebren Schaafsma, Giacomo Indiveri and Francky Catthoor, Stichting IMEC Nederland, High Tech Campus 31, Eindhoven 5656 AE, Netherlands; Institute of Neuroinformatics, University of Zurich and ETH Zurich, Switzerland; IMEC Leuven, Kapeldreef 75, 3001 Heverlee, Belgium
Heart activity can be monitored by means of ElectroCardioGram (ECG) measure which is widely used to detect heart diseases due to its noninvasive nature. Trained cardiologists can detect anomalies by visual inspecting recordings of the ECG signals. However, arrhythmias occur intermittently especially in early stages and therefore they can be missed in routine check recordings. We propose a hardware setup that enables the always-on monitoring of ECG signals into wearables. The system exploits a fully event- driven approach for carrying arrhythmia detection and classification employing a bio-inspired Spiking Neural Network. The two staged SNN topology comprises a recurrent network of spiking neurons whose output is classified by a cluster of Leaky-Integrate-and-Fire (LIF) neurons that have been supervisely trained to distinguish 17 types of cardiac patterns. We introduce a method for compressing ECG signals into a stream of asynchronous digital events that are used to stimulate the recurrent SNN. Using ablative analysis, we demonstrate the impact of the recurrent SNN and we show an overall classification accuracy of 95% on the PhysioNet Arrhythmia Database provided by the Massachusetts Institute of Technology and Beth Israel Hospital (MIT/BIH). The proposed system has been implemented on an event-driven mixed-signal analog/digital neuromorphic processor. This work contributes to the realization of an energy-efficient, wearable, and accurate multi-class ECG classification system.
This one because of AKIDA 2000:
1h: Spiking neural networks Monday, July 15, 5:30PM-7:30PM, Room: Duna Salon I, Chair: Federico Corradi 5:30PM A Spiking Network for Inference of Relations Trained with Neuromorphic Backpropagation [#19546] Johannes Christian Thiele, Olivier Bichler, Antoine Dupret, Sergio Solinas and Giacomo Indiveri, CEA/LIST, France; ETH Zurich and University of Zurich, Switzerland
The increasing need for intelligent sensors in a wide range of everyday objects requires the existence of low power information processing systems which can operate autonomously in their environment. In particular, merging and processing the outputs of different sensors efficiently is a necessary requirement for mobile agents with cognitive abilities. In this work, we present a multi-layer spiking neural network for inference of relations between stimuli patterns in dedicated neuromorphic systems. The system is trained with a new version of the backpropagation algorithm adapted to on-chip learning in neuromorphic hardware: Error gradients are encoded as spike signals which are propagated through symmetric synapses, using the same integrate-andfire hardware infrastructure as used during forward propagation. We demonstrate the strength of the approach on an arithmetic relation inference task and on visual XOR on the MNIST dataset. Compared to previous, biologically-inspired implementations of networks for learning and inference Monday, July 15, 5:30PM-7:30PM 29 of relations, our approach is able to achieve better performance with less neurons. Our architecture is the first spiking neural network architecture with on-chip learning capabilities, which is able to perform relational inference on complex visual stimuli. These features make our system interesting for sensor fusion applications and embedded learning in autonomous neuromorphic agents.
- Forums
- ASX - By Stock
- BRN
- 2020 BRN Discussion
BRN
brainchip holdings ltd
Add to My Watchlist
2.50%
!
20.5¢
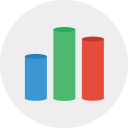
2020 BRN Discussion, page-854
Featured News
Add to My Watchlist
What is My Watchlist?
A personalised tool to help users track selected stocks. Delivering real-time notifications on price updates, announcements, and performance stats on each to help make informed investment decisions.
|
|||||
Last
20.5¢ |
Change
0.005(2.50%) |
Mkt cap ! $415.2M |
Open | High | Low | Value | Volume |
21.0¢ | 21.3¢ | 20.5¢ | $1.245M | 6.005M |
Buyers (Bids)
No. | Vol. | Price($) |
---|---|---|
11 | 1671491 | 20.5¢ |
Sellers (Offers)
Price($) | Vol. | No. |
---|---|---|
21.0¢ | 1345577 | 16 |
View Market Depth
No. | Vol. | Price($) |
---|---|---|
11 | 1671491 | 0.205 |
88 | 2193033 | 0.200 |
34 | 1010537 | 0.195 |
42 | 632437 | 0.190 |
8 | 341465 | 0.185 |
Price($) | Vol. | No. |
---|---|---|
0.210 | 1345577 | 16 |
0.215 | 874940 | 19 |
0.220 | 751291 | 21 |
0.225 | 618907 | 15 |
0.230 | 698793 | 21 |
Last trade - 16.10pm 02/04/2025 (20 minute delay) ? |
Featured News
BRN (ASX) Chart |