Hi 10,
This patent (US11151441 = US2018225562) relates to machine learning and is able to extract features of an image and label them autonomously. It builds on earlier BrainChip patents.https://worldwide.espacenet.com/patent/search/family/063037224/publication/US2018225562A1?q=us20180225562
... Principal computational approaches use either template matching with hand-designed features, reinforcement learning, or trained deep convolutional networks of artificial neurons and combinations thereof. Vector processing systems generate values, indicating color distribution, intensity and orientation from the image. These values are known as vectors and indicate the presence or absence of a defined object. Reinforcement learning networks learn by means of a reward or cost function. The reinforcement learning system is configured to either maximize the reward value or minimize the cost value and the performance of the system is highly dependent on the quality and conditions of these hand-crafted features. Deep convolutional neural networks learn by means of a technique called back-propagation, in which errors between expected output values for a known and defined input, and actual output values, are propagated back to the network by means of an algorithm that updates synaptic weights with the intent to minimize the error. The Deep Learning method requires millions of labelled input training models, resulting in long training times, and clear definition of known output values. However, these methods are not useful when dealing with previously unknown features or in the case whereby templates are rapidly changing or where the features are flexible. The field of neural networks is aimed at developing intelligent learning machines that are based on mechanisms which are assumed to be related to brain function. U.S. Pat. No. 8,250,011 describes a system based on artificial neural network learning. The system comprises a dynamic artificial neural computing device that is capable of approximation, autonomous learning and strengthening of formerly learned input patterns. The device can be trained and can learn autonomously owing to the artificial spiking neural network that is intended to simulate or extend the functions of a biological nervous system. Since, the artificial spiking neural network simulates the functioning of the human brain; it becomes easier for the artificial neural network to solve computational problems.
[0003] US20100081958 describes a more advanced version of machine learning and automated feature extraction using neural network. US20100081958 is related to pulse-based feature extraction for neural recordings using a neural acquisition system. The neural acquisition system includes the neural encoder for temporal-based pulse coding of a neural signal, and a spike sorter for sorting spikes encoded in the temporal-based pulse coding. The neural encoder generates a temporal-based pulse coded representation of spikes in the neural signal based on integrate-and-fire coding of the received neural signal and can include spike detection and encode features of the spikes as timing between pulses such that the timing between pulses represents features of the spikes.
[0004] However, the prior art do not disclose any system or method which can implement machine learning or training algorithm and can autonomously extract features and label them as an output without implementing lengthy training cycles. In view of the aforementioned reasons, there is therefore a need for improved techniques in spontaneous machine learning, eliminating the need for hand-crafted features or lengthy training cycles. Spontaneous Dynamic Learning differs from supervised learning in that known input and output sets are not presented, but instead the system learns from repeating patterns (features) in the input stream.
- Forums
- ASX - By Stock
- 2021 BRN Discussion
BRN
brainchip holdings ltd
Add to My Watchlist
2.44%
!
20.0¢
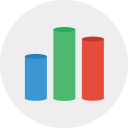
Hi 10, This patent (US11151441 = US2018225562) relates to...
Featured News
Add to My Watchlist
What is My Watchlist?
A personalised tool to help users track selected stocks. Delivering real-time notifications on price updates, announcements, and performance stats on each to help make informed investment decisions.
|
|||||
Last
20.0¢ |
Change
-0.005(2.44%) |
Mkt cap ! $405.0M |
Open | High | Low | Value | Volume |
20.5¢ | 20.5¢ | 20.0¢ | $406.0K | 2.012M |
Buyers (Bids)
No. | Vol. | Price($) |
---|---|---|
23 | 559479 | 20.0¢ |
Sellers (Offers)
Price($) | Vol. | No. |
---|---|---|
20.5¢ | 2087426 | 37 |
View Market Depth
No. | Vol. | Price($) |
---|---|---|
12 | 262016 | 0.200 |
38 | 2475083 | 0.195 |
74 | 2169655 | 0.190 |
49 | 1905401 | 0.185 |
71 | 1783990 | 0.180 |
Price($) | Vol. | No. |
---|---|---|
0.205 | 1851568 | 35 |
0.210 | 1146130 | 25 |
0.215 | 666226 | 19 |
0.220 | 1927120 | 45 |
0.225 | 534126 | 9 |
Last trade - 16.14pm 11/07/2025 (20 minute delay) ? |
Featured News
BRN (ASX) Chart |
The Watchlist
VMM
VIRIDIS MINING AND MINERALS LIMITED
Rafael Moreno, CEO
Rafael Moreno
CEO
SPONSORED BY The Market Online