You probably know that Brainchip's AKD1000 can perform regression analysis for vibration detection and analysis at 200 fps at less than 1 mW of power:
What you may have missed is that TATA Consulting Services one of Brainchip's research partners has been exploring the use of SNN/AKIDA technology for Computational Fluid Dynamics.Computational fluid dynamics (CFD) is a branch of fluid mechanics that uses numerical analysis and data structures to analyze and solve problems that involve fluid flows. Computers are used to perform the calculations required to simulate the free-stream flow of the fluid, and the interaction of the fluid (liquids and gases) with surfaces defined by boundary conditions. With high-speed supercomputers, better solutions can be achieved, and are often required to solve the largest and most complex problems. Ongoing research yields software that improves the accuracy and speed of complex simulation scenarios such as transonic or turbulent flows. Initial validation of such software is typically performed using experimental apparatus such as wind tunnels. In addition, previously performed analytical or empirical analysis of a particular problem can be used for comparison. A final validation is often performed using full-scale testing, such as flight tests.CFD is applied to a wide range of research and engineering problems in many fields of study and industries, including aerodynamicsand aerospace analysis, hypersonics, weather simulation, natural science and environmental engineering, industrial system design and analysis, biological engineering, fluid flows and heat transfer, engine and combustionanalysis, and visual effects for film and games
2. Stabilization and Acceleration of CFD Simulation by Controlling Relaxation Factor Based on Residues: An SNN Based Approach
Mithilesh Maurya, Dighanchal Banerjee, Sounak Dey, Dilshad Ahmad
TCS Research, India
{mk.maurya|dighanchal.b|sounak.d|dilshad.ahmad}@tcs.com
Abstract
Computational Fluid Dynamics (CFD) simulation involves the solution of a sparse system of linear equations. Faster convergence to a physically meaningful CFD simulation result of steady-state physics depends largely on the choice of optimum value of the under-relaxation factor (URF) and continuous manual monitoring of simulation residues. In this paper, we present an algorithm for classifying simulation convergence (or divergence) based on the residues using a spiking neural network (SNN) and a control logic. This algorithm maintains optimum URF throughout the simulation process and ensure accelerated convergence of the simulation. The algorithm is also able to stabilize and bring back a diverging simulation to the converging range automatically without manual intervention. To the best of our knowledge, SNN is used for the first time to solve such complex classification problem and it achieves an accuracy of 92.4% to detect the divergent cases. When tested on two steady-state incompressible CFD problems, our solution is able to stabilize every diverging simulation and accelerate the simulation time by at least 10% compared to a constant value of URF.
1 Introduction
System of linear equations governing the model of solid-fluid interactions in Computational Fluid Dynamics (CFD) domain are usually solved using iterative methods [6, 19, 7] to reach to an acceptable numerical solution. This iterative process is time consuming, compute intensive, and the solution does not guarantee convergence to a physically meaningful solution for a given set of simulation parameters. This results into huge waste of man hours and computation resource. Though controlling factors such as under relaxation factor (URF) and convergence indicators such as simulation residue history (SRH) are tuned to ensure convergence, but it requires manual intervention to select suitable values. Thus, an automatic monitoring mechanism of residue history (to interpret convergence or divergence) and a subsequent control logic to auto-tune the URF would help in: (i) stabilising a diverging simulation, (ii) reaching faster convergence by accelerating converging simulation.
Fuzzy logic based approaches [15, 3] and Expression based methods [12, 20] have been applied to calculate & control URF but they tend to increase the computation time and are not applicable across all classes of CFD problems. RL based acceleration methods [13] for CFD simulations are computationally expensive and slow. Looking at the compute-intensive nature of simulation and to cater the requirement of early detection of divergence from as less data as possible, use of Spiking Neural Networks (SNN), a 3rd generation ML framework inspired from functionalities of mammalian brain, can be a good choice to reach to a useful solution [14]. SNNs are comparatively faster to learn from sparse data and are far less compute & power intensive. SNNs achieve this through asynchronous event handling and co-location of memory and computation [1, 4, 22].
Machine Learning and the Physical Sciences workshop, NeurIPS 2022.
In this paper, we propose an improved and robust solution to stabilize diverging CFD simulations and accelerate the converging CFD simulation by keeping the URF to its optimum value. The residual value over time is treated as a continuous time series. Current data of a fixed size window from this time series is passed to the SNN to be classified either as diverging or as converging. Depending on the classification, a control logic is used to change the URF. The classifier and control logic work together without interfering with the CFD simulation i.e. the process is non-invasive and works in parallel to the simulation. We demonstrate the efficacy of our approach via two steady-state incompressible CFD problems namely Backward Facing Step and Flow inside Tundish. We verified our findings by running the algorithm with OpenFOAM [18] and Ansys Fluent [11] simulators. We found that (i) the SNN is able to detect the divergent cases with 92.4% accuracy (with window size = 30), (ii) our solution is able to stabilize each and every diverging simulations and finally, (iii) it is also able to accelerate the simulation time by at least 10% compared to a fixed URF value…
Conclusion and Future Works. Our proposed solution is generic and can be integrated with any CFD tools. This automated process saves a lot of manual effort and time thereby making it beneficial for many industry scale CFD problems that run for days. Moreover, the SNN model can easily be retrained with additional data to improve the accuracy and to cater to different classes of problems. In future, we intend to improve the existing fixed control logic to an adaptive URF controller varying with the SR value. We also intend to test the performance and power consumption of the SNN by running it on a real neuromorphic hardware such as Intel Loihi [2, 9] or Brainchip Akida [1].
This research paper was published by TCS in 2022 and as we know in 2023 TATA Elxsi and Brainchip partnered to drive the adoption of AKIDA technology into medical and industrial use cases. I would suggest that given the fact that AKIDA technology has proven itself in regression analysis that it is highly likely that TCS did proceed to prove out the use of AKIDA technology for Computational Fluid Dynamics and this was part of the weight of evidence that led to TATA Elxsi deciding to partner with Brainchip.
While there is no evidence to suggest that Brainchip is involved the following link is to one interesting application of Computational Fluid Dynamics:
https://www.autoevolution.com/news/airbus-to-develop-jet-wings-that-can-adjust-their-shape-automatically-in-flight-170010.html
My opinion only DYOR
Fact Finder
- Forums
- ASX - By Stock
- BRN
- BrainChip Boosts Space Heritage with Launch of Akida into Low Earth Orbit
BRN
brainchip holdings ltd
Add to My Watchlist
10.3%
!
21.5¢
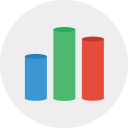
BrainChip Boosts Space Heritage with Launch of Akida into Low Earth Orbit, page-6
Featured News
Add to My Watchlist
What is My Watchlist?
A personalised tool to help users track selected stocks. Delivering real-time notifications on price updates, announcements, and performance stats on each to help make informed investment decisions.
|
|||||
Last
21.5¢ |
Change
0.020(10.3%) |
Mkt cap ! $435.4M |
Open | High | Low | Value | Volume |
19.5¢ | 21.8¢ | 19.5¢ | $2.572M | 12.25M |
Buyers (Bids)
No. | Vol. | Price($) |
---|---|---|
12 | 670955 | 21.0¢ |
Sellers (Offers)
Price($) | Vol. | No. |
---|---|---|
21.5¢ | 200777 | 9 |
View Market Depth
No. | Vol. | Price($) |
---|---|---|
11 | 665955 | 0.210 |
17 | 1094375 | 0.205 |
29 | 1517289 | 0.200 |
20 | 701683 | 0.195 |
66 | 2349375 | 0.190 |
Price($) | Vol. | No. |
---|---|---|
0.215 | 200777 | 9 |
0.220 | 2201175 | 52 |
0.225 | 1813631 | 21 |
0.230 | 1091664 | 27 |
0.235 | 413848 | 15 |
Last trade - 16.18pm 01/07/2025 (20 minute delay) ? |
Featured News
BRN (ASX) Chart |
The Watchlist
RC1
REDCASTLE RESOURCES LIMITED
Ron Miller, Non-Executive Director
Ron Miller
Non-Executive Director
Previous Video
Next Video
SPONSORED BY The Market Online